Industry Bio | Academic Bio
As a Human-AI Interaction specialist with a Ph.D. from Carnegie Mellon University (CMU), I focus on developing interactive technologies that enhance cognitive efficiency and creativity in human-AI collaboration. My research aims to address real-world challenges across domains including scientific discovery, engineering, product innovation, and design thinking. With years of experience, my expertise spans:
- • Design, development, and deployment of ML models and LLM-infused full-stack interactive systems
- • Data collection and analysis pipelines, including bespoke crowdsourcing systems and NLP techniques
- • Design and execution of quantitative, qualitative, and mixed-method evaluations at various scales (e.g., case, laborotory, and field studies)
My work has led to tangible real-world impacts, including improving engagement with personalized email alerts for scientific research papers, benefiting thousands of users (deployed at the Allen Institute for Artificial Intelligence) and contributing to research allocating $2M in prize money for global conservation innovation contests (organized by Conservation X Labs, a conservation-focused non-profit).
Throughout my work, I've collaborated with professionals across various disciplines (e.g., engineers, designers, scientists, and teachers) and organizations (e.g., Toyota Research Institute, Conservation X Labs, Allen Institute for AI, MIT). My contributions have been recognized with a Best Paper Award at ACM CHI 2024, a Best Paper Honorable Mention Award at CHI 2025, and a Google Cloud Innovator Award (2021). Additionally, my research findings have become required reading at Virginia Tech and CMU.
Hyeonsu Kang (Ph.D., Carnegie Mellon University) is a Human-AI Interaction researcher specializing in enhancing cognitive efficiency and creativity through collaborative AI systems. His work combines cognitive theories with cutting-edge AI to improve problem-solving and ideation for both experts and novices across various domains. Kang's research spans three key areas:
- 1. Enhancing outside-the-box thinking for domain experts and novices [TOCHI'22, CHI'22, NAACL'22, CHI'25 🥇 & NeurIPS'23 & CHI'24, AAAI'24]
- 2. Facilitating effective discovery and synthesis of relevant prior knowledge [UIST'23, CHI'23, UIST'22, CHI'22, CHI'24]
- 3. Promoting social learning and idea development through augmented feedback and expertise exchange [CHI'18, UIST'17, Collective Intelligence'19, CHI'24 🏆]
Kang's contributions have been recognized with a best paper award at ACM CHI 2024, a best paper honorable mention award at ACM CHI 2025, and a Google Cloud Innovator Award (2021). His work has been published in premier NLP and HCI conferences and journals, including ACM CHI, UIST, TOCHI, AAAI, NAACL, and NeurIPS. His research has been funded by the National Science Foundation, the Allen Institute for Artificial Intelligence, the Office of Naval Research, the Toyota Research Institute, and Google Cloud. Prior to his Ph.D., Kang received his BS in Computer Science and Engineering at Seoul National University. He also worked and interned at MIT, the Allen Institute for AI, UC San Diego, and Tableau Software. He was previously supported by the South Korean National Scholarship for Science and Engineering.
Publications
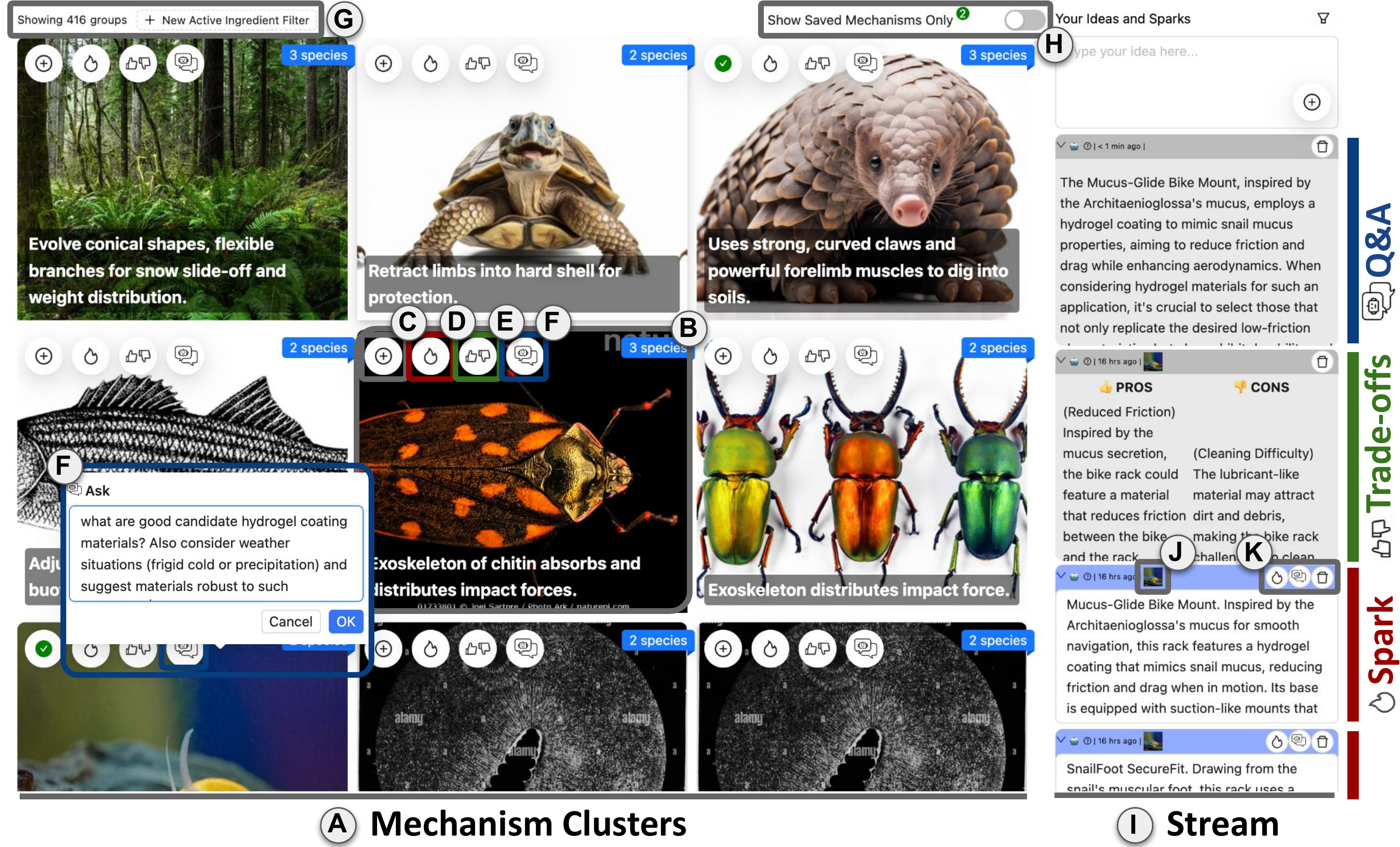
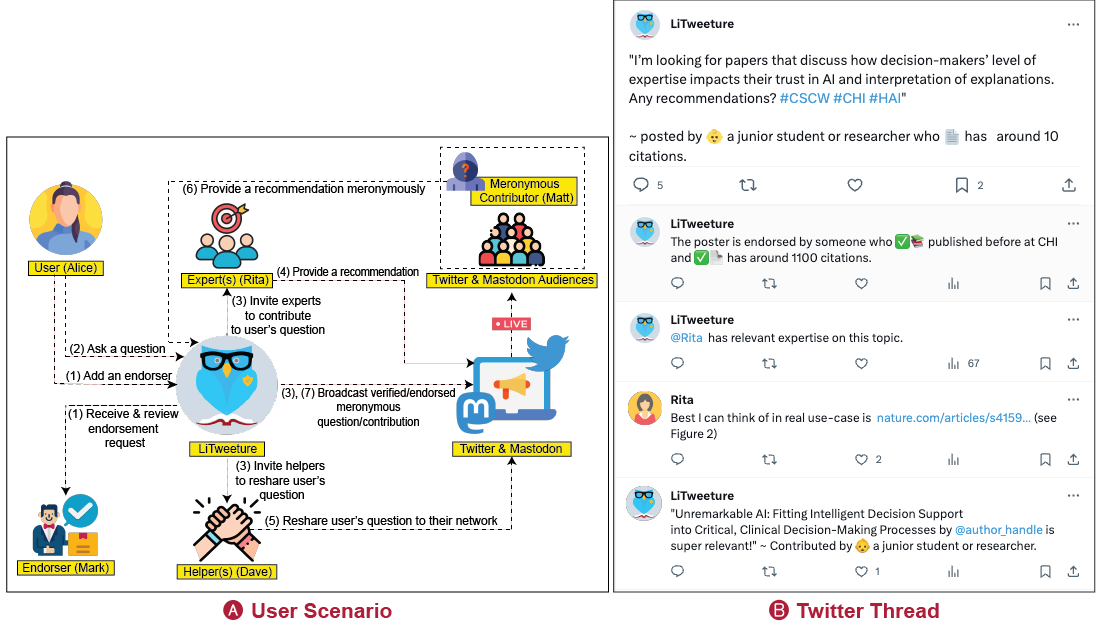
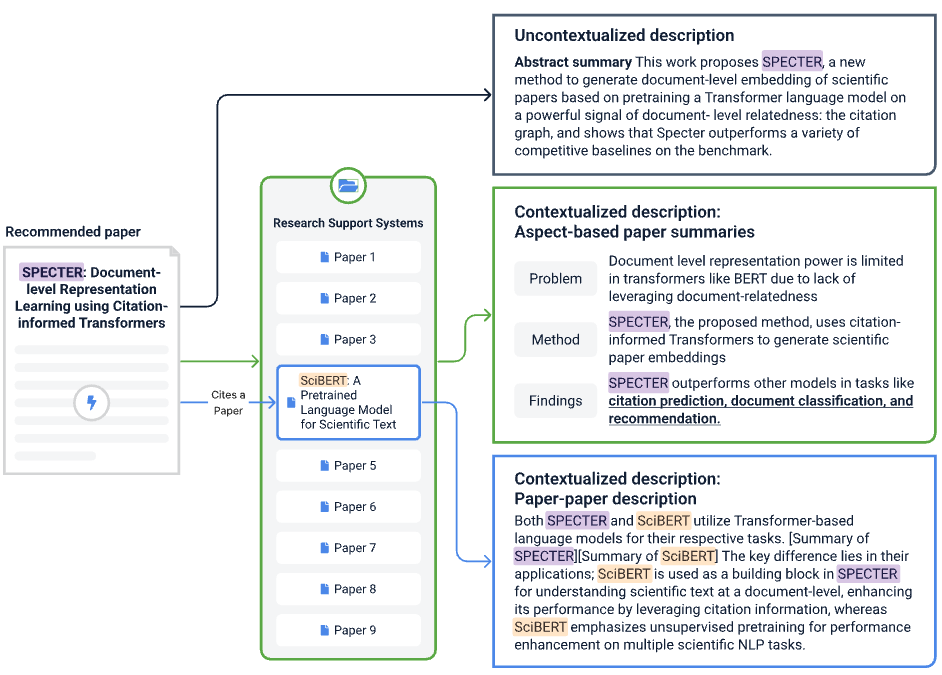
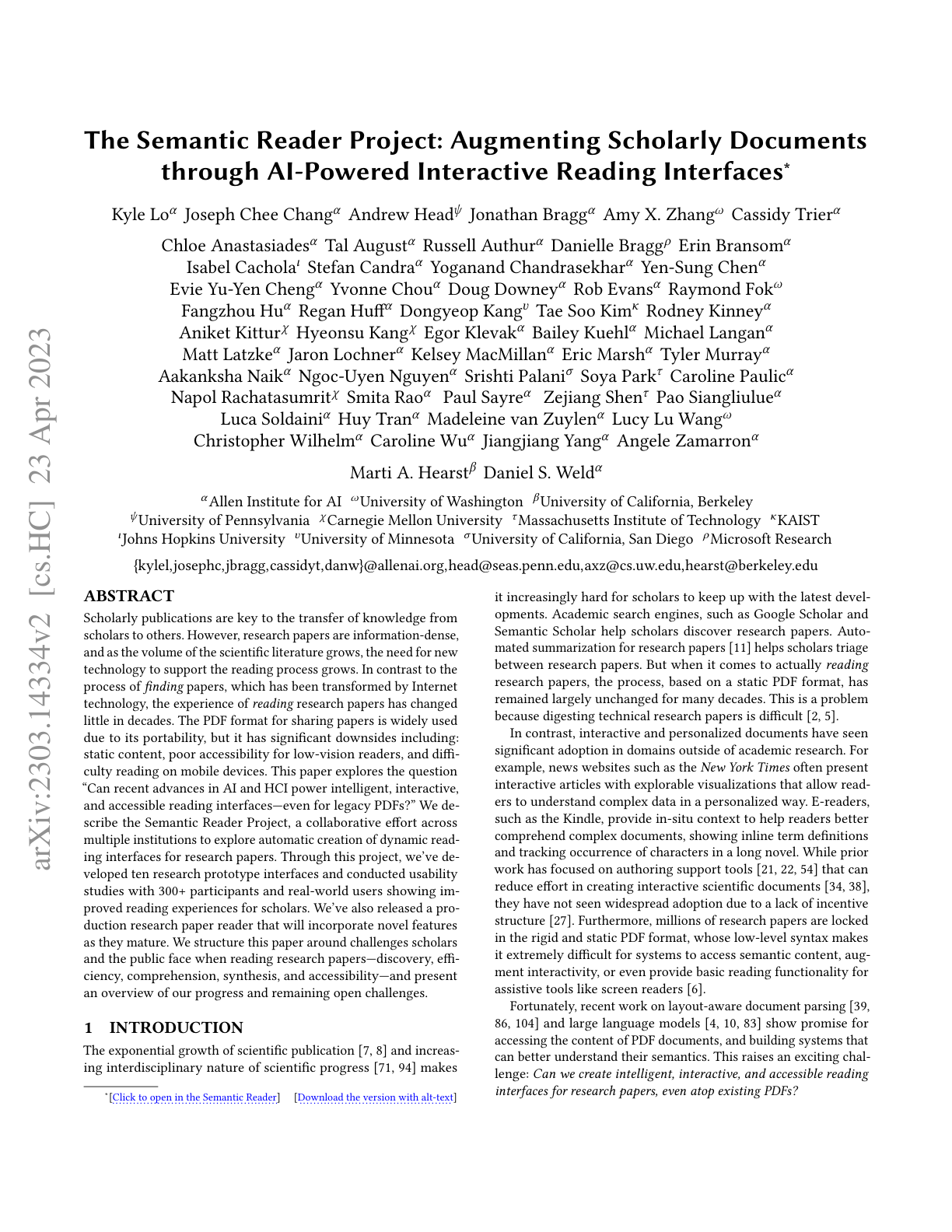
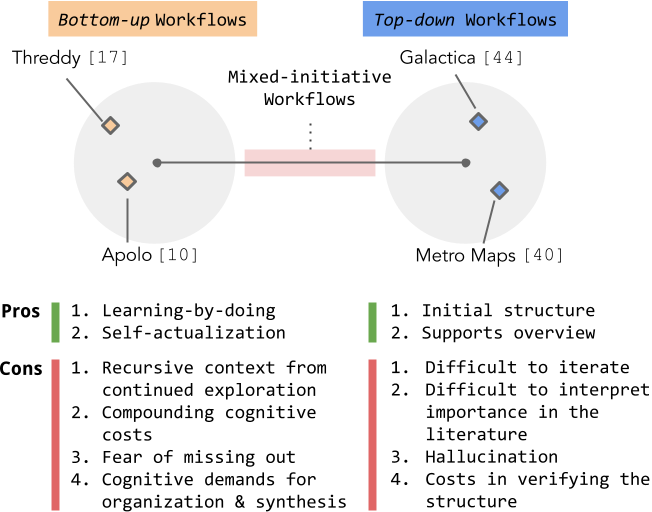
A demo video of Synergi is available here.
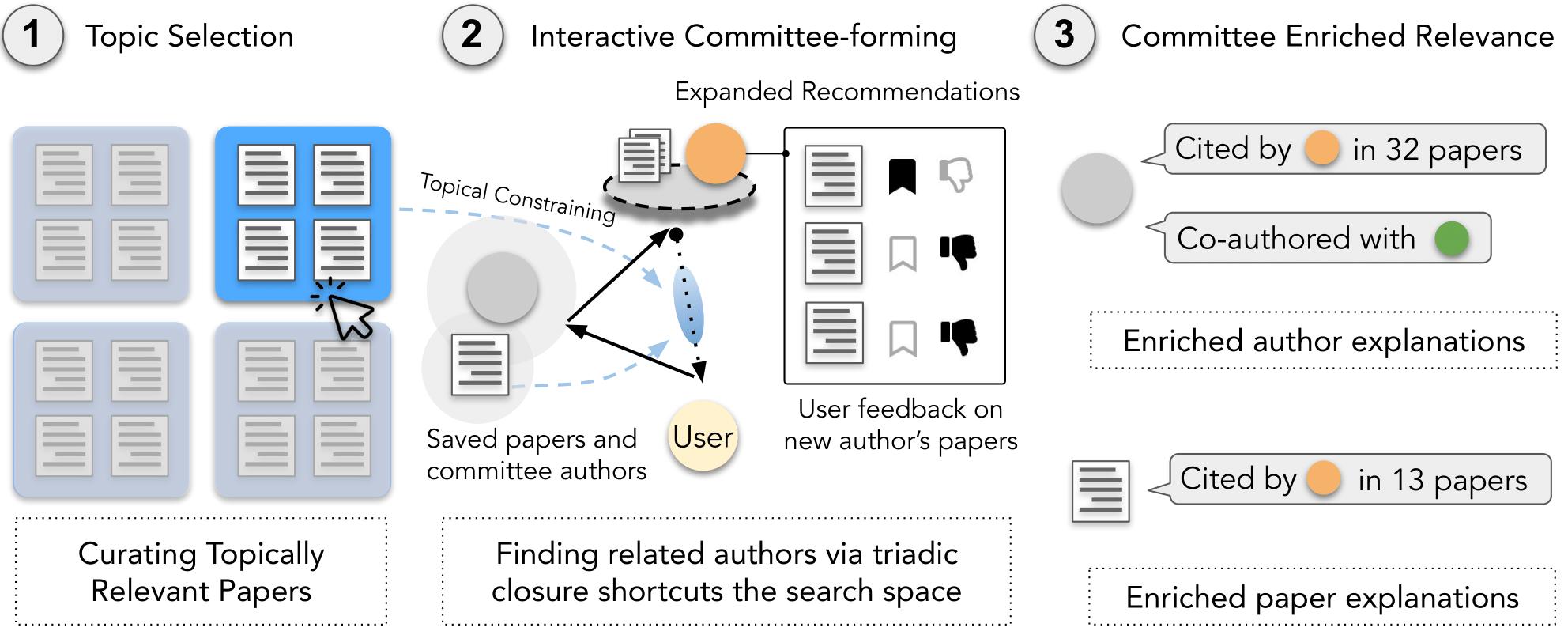
A demo video of ComLittee is available here.
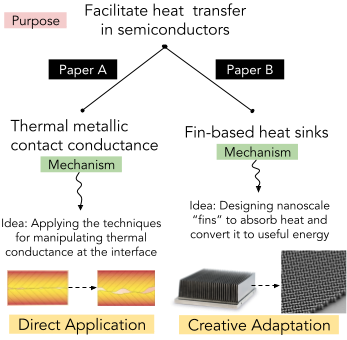
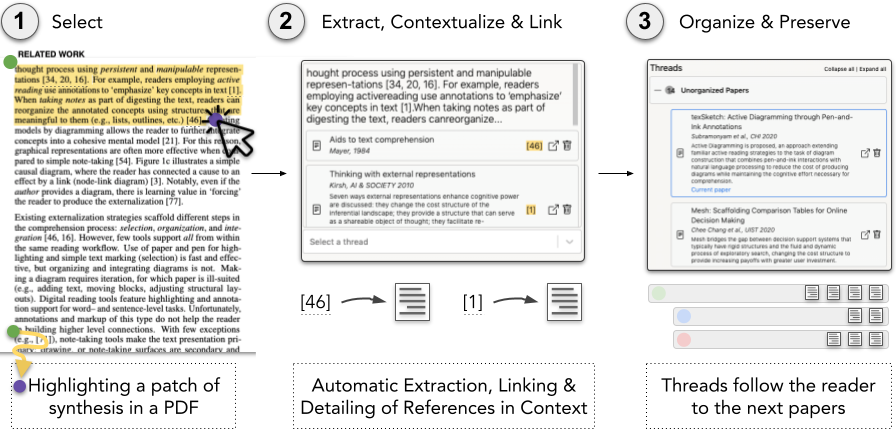
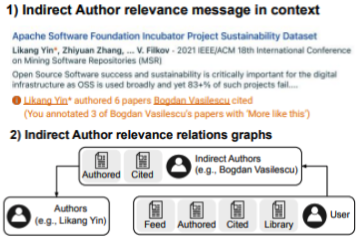
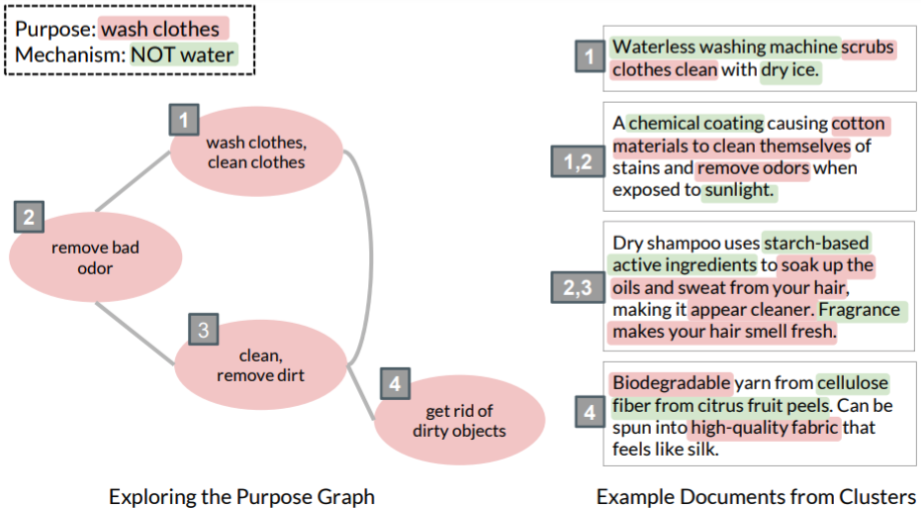
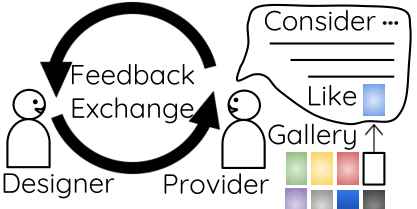
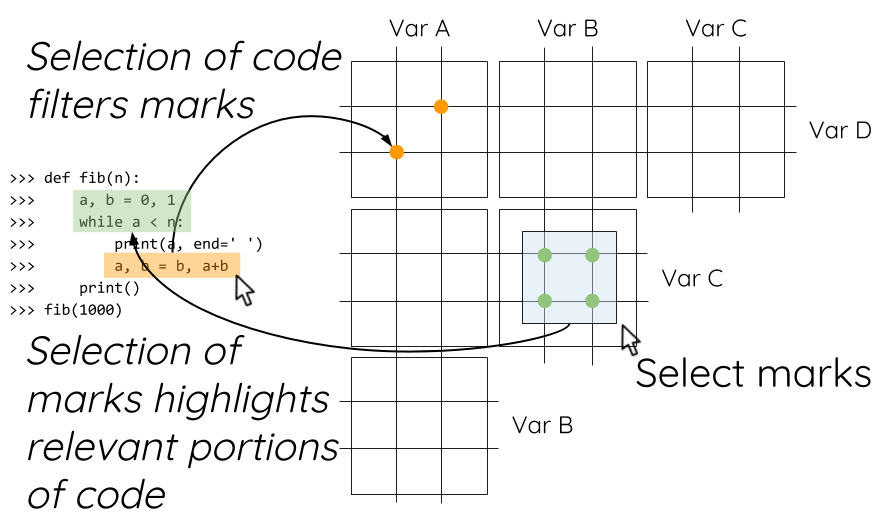
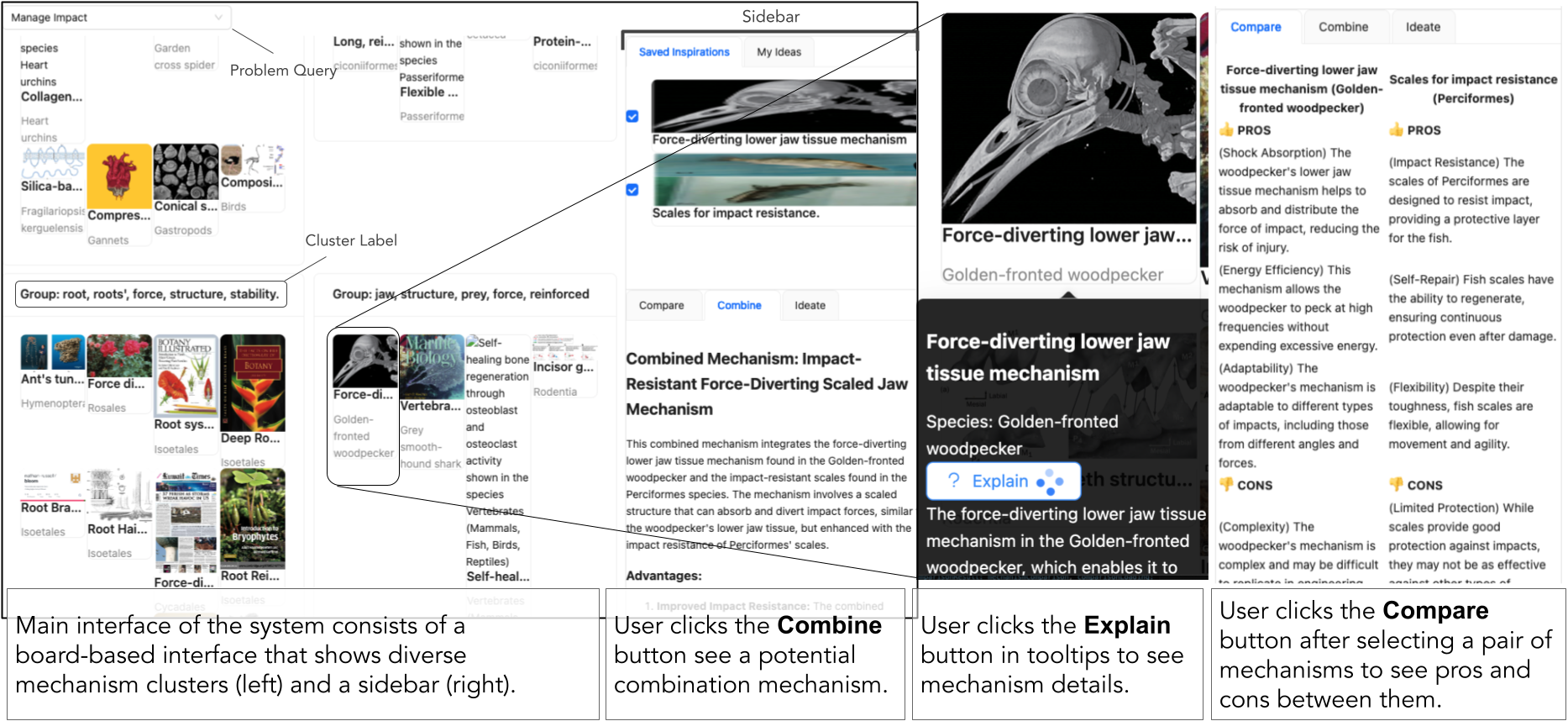
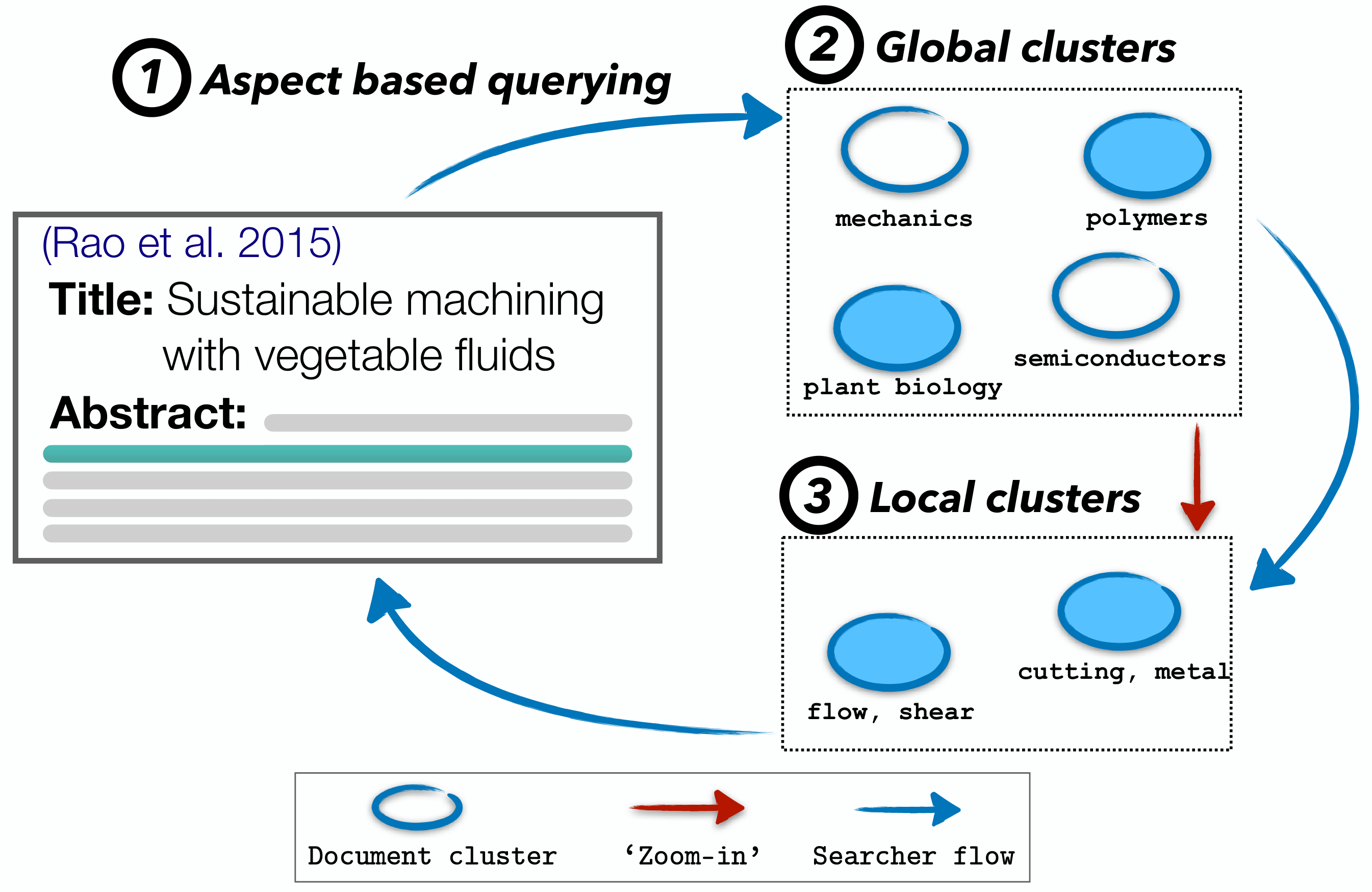
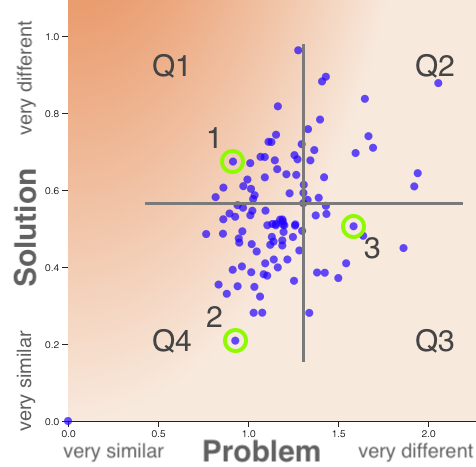
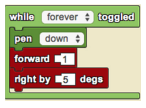